Volpe-Developed Algorithms Help in Mining Large Driver Datasets
Researchers who conduct naturalistic driving studies to understand driver performance face the proverbial needle-in-the-haystack problem. Cars outfitted with sensors and video cameras record driver behavior and road and traffic conditions, providing massive amounts of naturalistic data. To shed light on driver safety, researchers must comb through huge datasets to locate the safety-critical events. With datasets that exceed the volume of data housed in the U.S. Library of Congress, finding the needle can be daunting.
Consider the Safety Pilot Model Deployment, a year-long Connected Vehicle field test taking place in Ann Arbor, MI led by the Intelligent Transportation Systems Joint Program Office. Approximately 3,000 cars, trucks, and buses are participating in the field test, and Volpe researchers estimate that 28 million miles of driving data will be collected. Vehicles are outfitted with safety technologies such as forward collision warnings and lane change crash warnings, as well as a suite of sensors and video cameras. Volpe has the challenging task of combing through the large dataset to assess safety benefits, driver acceptance, and system capabilities.
"It's all about mining the data efficiently," said Emily Nodine, a mechanical engineer in Volpe's Center for Advanced Transportation Technologies. Nodine and her colleagues use a series of Volpe-developed algorithms to identify events of interest in the massive dataset. According to Nodine, "the safety benefits methodology that we use was developed by Volpe. The basic approach is similar for each field test, but the algorithms always need be tweaked to accommodate each individual dataset."
Volpe's safety benefits methodology looks at situations in which drivers get into safety-critical events, which are situations in which drivers intervene to avoid crashes. To identify these events, engineers run algorithms to determine, for example, if the vehicle is accelerating, maintaining speed, or decelerating. Rapid acceleration and deceleration may signal a safety-critical event. Volpe engineers then use their video analysis tool to discover, for example, how close a driver got to the lead vehicle before slamming on their brakes, what events led up to the event and the extent to which technology helped the driver.
Statistical analyses are then performed on these safety-critical events, which provide information on how the technology impacts driver behavior and ultimately helps drivers avoid crashes. As a result of the Volpe-developed algorithms, Volpe's engineers are able to make informed decisions about the effectiveness of the technologies being evaluated.
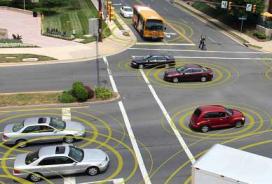
The Safety Pilot Model Deployment for DOT's connected vehicle program has yielded more data than is housed in the entire U.S. Library of Congress. (U.S. Department of Transportation photo)